Search or browse our knowledge base for information on AI, human values at risk, case studies, policy & standards around the globe and AIFCS advocacy.
An AI Harms and Governance Framework for Trustworthy AI
Trustworthy AI
The driver for trustworthy AI systems is the belief that their development, deployment and use will accelerate when society trusts them, leading to greater economic prosperity and human flourishing.
Trust is a fundamental component of societies but is based on trust between people rather than trust in artifacts. We may feel confident driving over a bridge because ultimately, we are putting our trust in the designers, builders and the regulators that it has been designed and built correctly and that materials have been checked along the way. This trust can be broken down when accidents occur because someone has not done their job properly, has cut corners or failed to heed warnings of structural deficiencies or erosion.
Trust in the context of AI is trust in the designers, companies and deployers of an application, that the application will do the job for which it has been created, correctly, reliably – that is produce the same result time and time again, without prejudice and will not do me any harm and that my experience of it will be beneficial. Our use of such applications will therefore entail a willingness to take risk – to trust the actors behind the design and deployment. Trust may be built over time as a result of repetitive use of an artifact where we build confidence that it does the job it was designed to do without harming us.
Because of their nature, the full benefit of these technologies will be attained only if they are aligned with our defined values and ethical principles. We must therefore establish frameworks to guide and inform dialogue and debate around the non-technical implications of these technologies.
IEEE Ethically Alligned Design, 2019
Ethics for all
Over eighty bodies around the world have developed ethics guidelines for AI. Jobin provides a comprehensive survey of these guidelines and a summary of the ethical principles common to all is shown below, adapted from Jobin.
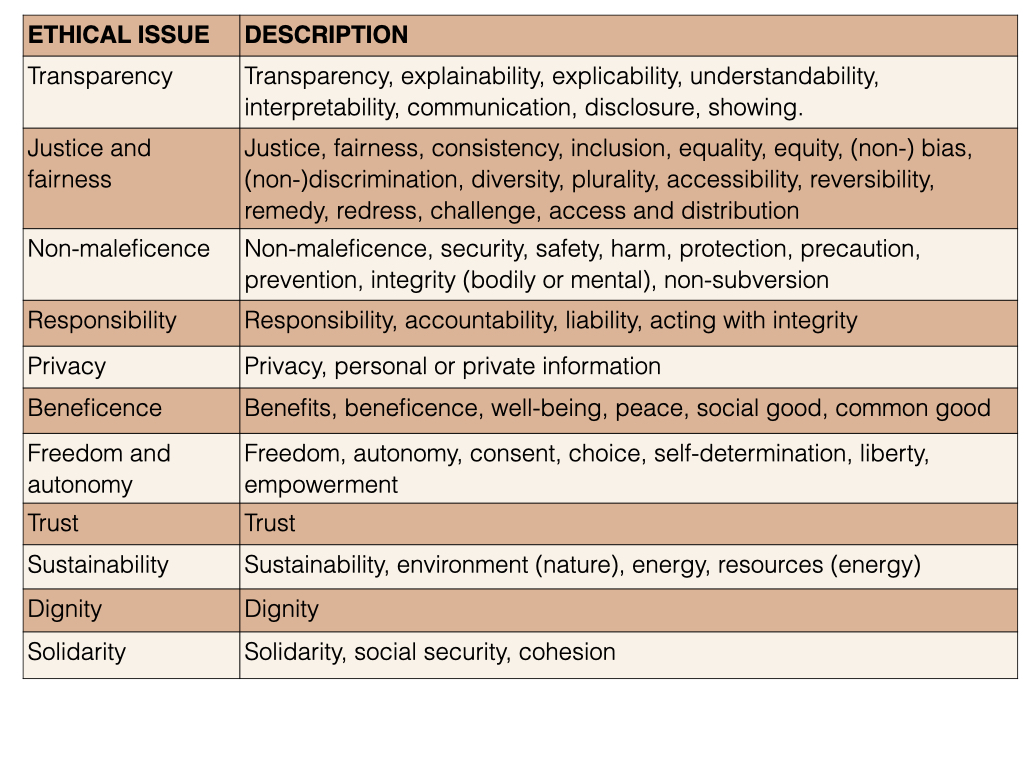
There’s a good deal of overlap between the various reports and recommendations, with the importance of AI being for the common good standing out in most, alongside AI not harming people or undermining their human rights. Human rights are often the basis on which the idea of human autonomy is founded and relates to individual freedoms as well as the right to self-determination.
problematic use cases are predictive policing, social credit scores, facial recognition and conversational bots.
Centre for European Policy Studies (CEPS)
The key ethical principles surrounding the use of AI systems in the table above, with the exception of beneficence, reflect the potential for harm in ways other than purely physical. The challenge with such principles is that they lack specificity and are used to convey a sense of what AI systems should or should not do to users. Some, like Transparency, become the focus for ethical concerns and require a separate Ethical Risk Assessment that can lack specificity.
A Taxonomy of Harms to Humanity
A different approach uses a taxonomy of AI applications against which a more specific set of harms to humanity are defined as shown in the table below (adapted from J. Peckham, Masters or Slaves?). These seek to be more descriptive of the harms that can be caused to users of each particular use case of an AI system in the taxonomy.
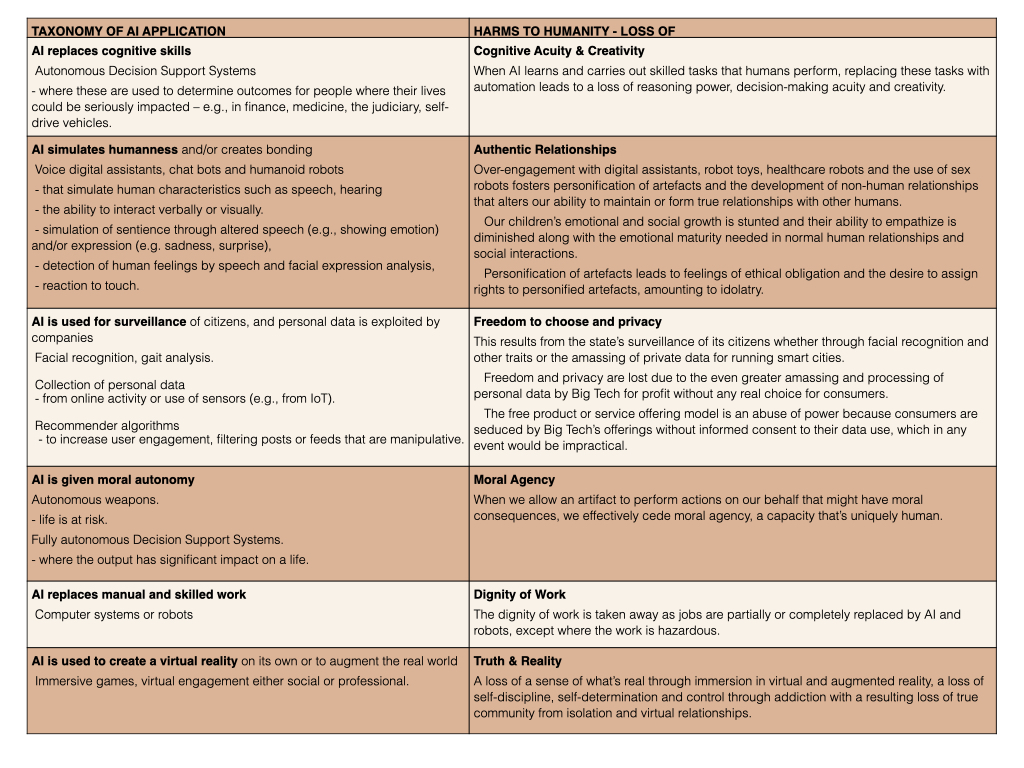
A Framework for Trustworthy AI
The IEEE P7001 Standard on Transparency for Autonomous Systems approaches trust as a governance issue by creating five levels of transparency requirements for each of five different stakeholders. These are used to determine the level of transparency required (level 0-5) and the compliance for each stakeholder of any autonomous application. Explainability is regarded as a subset of transparency and relates to the extent to which information is accessible to non-experts.
In a similar way, other ethical concerns listed in the table above adapted from Jobin, such as accountability and justice (also termed fairness) can be used to create a set of governance principles, each working together to provide an holistic framework to promote trust.
The definition and implementation possibilities for each of the governance principles or pillars is shown in the figure below.
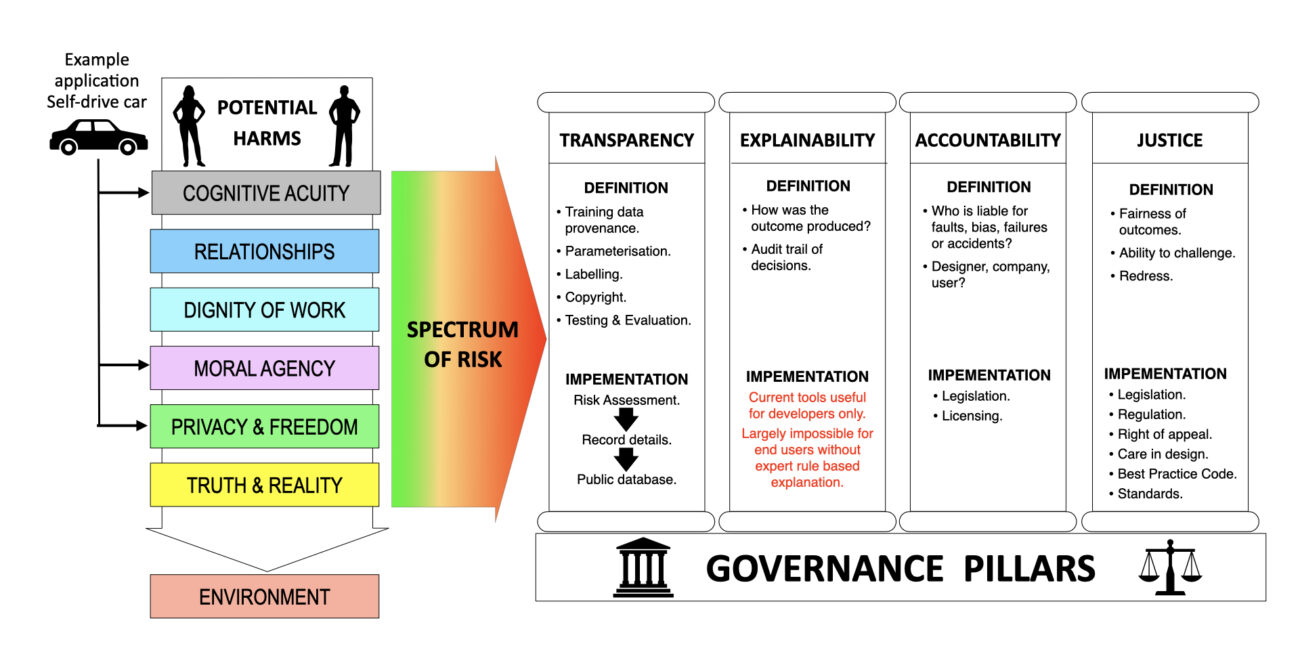
Transparency
Transparency is used by policy makers in a variety of ways from a simple data base recording the provenance of data sets, ownership and responsibility for algorithmic tools and impact assessments [9], to an ability for the output of the algorithm to be explained or its decision process uncovered [10].
The monitoring and use of data to continue model improvement in speech recognition for products like Amazon’s Alexa, has caused public concern over data privacy. Whilst the human inspection and labelling of such data is enormously helpful to the developers and improves accuracy, the trade-off is the loss of privacy.
This clearly raises tensions between improving model performance and respecting users’ privacy. The use of training data, both to initiate the model, and subsequent use of data to improve the model thus becomes a governance issue that cannot be resolved technically but is itself an ethical issue that will require regulation, perhaps in ways similar to Europe’s General Data Protection Regulation (GDPR). Were such data to be unavailable by law without explicit informed consent, then further improvement in such algorithms would be slow, expensive and potentially limited by a lack of real-world data.
A requirement to register such details about an application would provide transparency to users and regulators and is one part of the process in protecting privacy. The accountability and justice component of governance may require the implementation of privacy laws to protect users’ privacy where it is declared that data harvesting is carried out, for example in social media platforms. Where such laws exist, a transparency audit will reveal breaches (provided it is a regulatory requirement to file records).
Explainability problematic
Given the inherent difficulties of explaining the actions of stochastic AI algorithms, explainability in this paper addresses to what extent an AI algorithm can explain its actions or output and is the place to capture the risks associated with any unexplainable output. This is particularly relevant in applications that can cause harm because their output cannot be explained. On the flip side, recent developments in Generative AI, especially where natural language is output, are potentially harmful where they give the impression that the output can be explained to a non-expert user. Whether information is understandable by a non-expert is not the same as whether an algorithm can explain its output. In the case of Generative AI, even experts seem to be unable to explain so called “hallucinations” although it ought to be self-evident that a stochastic process could produce unexplainable output.
A conclusion from this consideration is that in applications where people’s lives are impacted by an AI systems output, such as an Automated Decision Support System (ADSS), there should always be a right to request human analysis or even an opt out option. This dimension of governance can thus be used to explicitly flag up a need for specific legislation in the Justice dimension, thus providing a holistic framework for trustworthy AI.
Accountability
Accountability for faults, failures or harms arising out of the use of AI systems is a matter of legislation, that will require regulators and politicians to act. Self-drive or autonomous vehicles provide an example. In the U.K., the Independent Law Commission has proposed a new system of legal accountability [16] where “the person in the driving seat would no longer be a driver but a “user in charge”.” This person would no longer be liable for offences that arise from the driving task. Responsibility would pass to an “Authorised Self-Driving Entity (ASDE) and in an authorised fully self- driving vehicles, people would become passengers, putting the licenced operator in charge and responsible.”
Accountability in another application type, digital assistants, illustrates the challenges arising from the use of Natural Language Processing (NLP) systems such as deployed in Alexa. In a widely reported story [17], a ten year old child asking Alexa for a “challenge to do” received the reply “Plug in a phone charger about halfway into a wall outlet, then touch a penny to the exposed prongs.” Fortunately, the child didn’t carry out the challenge, but what if she had and who would have been liable if she had been electrocuted? The nature of such NLP systems and the fact that they have no understanding of the answers that they give highlights why accountability becomes a much more complex matter because the outputs of such devices cannot be predicted and additional ethical questions need to be asked to determine what, if any, governance concerns should be addressed, as we shall see later in the orthogonal harms dimension of my framework.
Justice
One of the most publicised aspects of unfair or unjust AI systems relates to biased data sets used for training ML algorithms, giving rise to discrimination. Obermeyer, for example, has pointed out in [18] that black patients were discriminated against in an AI system widely used in US hospitals. This was due to using the money being spent on patients as a proxy for health needs. On average, black people consume less resources, thus biasing the data when using this parameter as a proxy for health needs. This example is an obvious case where it is clear that improvements in design could be made to find better indicators of health need that reduce, if not eliminate, discrimination.
A major challenge, however, in data sampling and removing bias lies in determining the criteria to be used.
Who determines what an unbiased data set looks like, given that ML is simply modelling on historic data as a proxy for the reasoning and abduction that humans carry out?
Jeremy Peckham IEEE Computer Magazine,March 2024
Humans themselves are biased and this bias is encapsulated in historic data, often used for training. Using algorithmic methods or human screening simply introduces another form of bias into the data. Historic patterns of crime, as an example, might simply be a starting point in human evaluation but if crime predominantly occurs amongst a particular socio-economic group, trying to balance the data set in some way will itself introduce another bias. What is regarded as social bias is a concept that is not fixed in any given society and can change over time. It also differs between different groups and ideologies.
The process of uncovering bias is an iterative one that requires reasoning, empirical evidence, abduction and value judgement. In the final analysis, justice needs to be served through a right of appeal to human adjudication, rather than relying on a black box.
Data bias can also occur from malicious bias. An independent data audit through a Distributed Ledger Technology (DLT) is proposed by Thiebes in [19] and has the benefit of at least identifying malicious bias or intent. This approach is already being trialled to secure the provenance of news and images. Safe.Press, a news certification service developed by Block Expert in France uses blockchain technology to combat fakenews. The Content Authority Initiative with partners including Adobe, Twitter and the BBC is another venture seeking to address the problem using technology developed by start-up company, TruePic. TruePic also uses blockchain technology but is developing a more scalable system using public/private keys based on a Public Key Infrastructure.
At best, just outcomes could be improved through careful design, data sampling and labelling, but it is naïve to assume that data bias can be eliminated and that a data set could ever be complete.
Human rights and human well-being should not be held as trade-offs, with one to be prioritized over the other. In this regard, well-being metrics can be complementary to the goals of human rights, but cannot and should not be used as a proxy for human rights or any existing law.
IEEE Ethically Alligned Design, 2019
An Holistic Ethical Framework to promote Trust
The four governance pillars are not separated from the other ethical concerns but become part of the framework for implementing measures that will promote trust. In the framework proposed here, the ethical concerns shown in the adapted table form Jobin are mapped into an orthogonal taxonomy of AI applications and specific harms to users (see Taxonomy table above) that exist on a spectrum of risk. In a similar way, the application of IEEE P7001 [8] requires an Ethical Risk Assessment to identify the degree of transparency required for a particular application.
A Spectrum of Risk
For any given use case of AI there exists a spectrum of risk to the six key human values. Where an application presents risk to more than one of the key values, the risks for each needs to be determined independently.
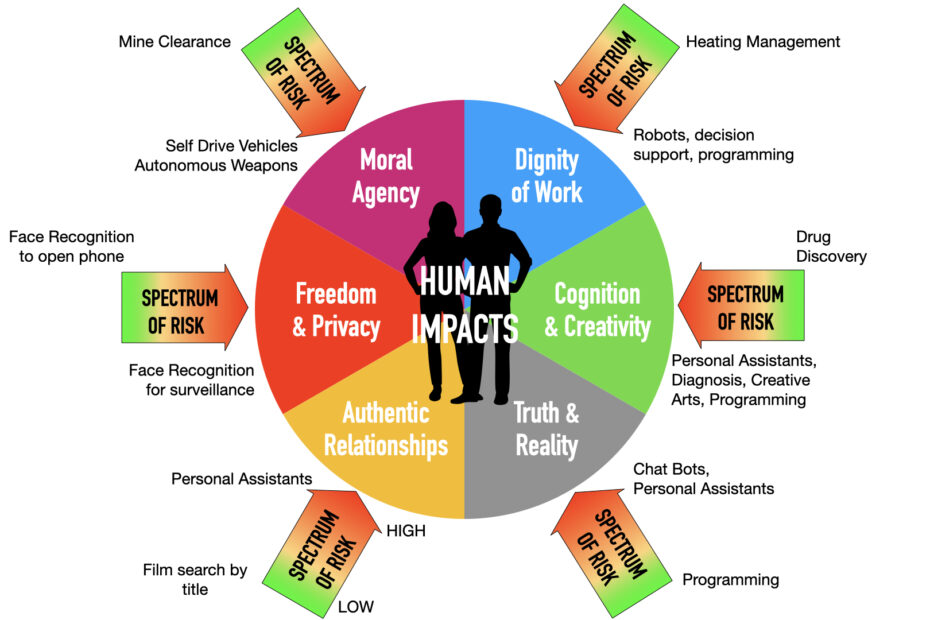
The assessment of Transparency requirements in IEEE P7001 is required for each of a number of defined stakeholders such as end users, safety inspectors and lawyers. Applying such an approach to the Transparency pillar adds depth to the proposed framework and could also be the focus for assessing the risks for each stakeholder.
Mapping ethics into action
Mapping ethical issues into a taxonomy of specific harms and a set of orthogonal governance principles allows a much sharper focus for evaluating any particular application of AI. For each application it is possible to assess each of the potential harms and the level of risk through the governance process, starting with transparency and explainability. These assessments then inform the more legal aspects of governance providing more specificity on what legislation and compliance with standards might be required. The governance axis therefore becomes the main focus for implementation, based around the risk assessment of the harms in a particular use case in the taxonomy (see table above). It also highlights the inherent limitations of algorithmic and data selection solutions to the explainability and justice dimensions of governance that will require human intervention and oversight in ensuring trustworthiness.
Multiple harms possible for a given use case
The potential for subjectivity in assigning an application to a particular risk category in this taxonomy might be seen as problematic, especially in cases where an application might fit into different categories. In this framework, each category of harm should be considered, initially in the Transparency pillar of governance, and the risk assessed. A self-driving vehicle therefore would be assigned to several potential harm categories, such as replacing cognitive skills, used for surveillance (sensor data monitored by government or a company) and given moral agency. Each represents different risks but each needs to be considered.
Self-drive vehicle example
A self-driving vehicle therefore would be assigned to several potential harm categories, such as replacing cognitive skills, used for surveillance (sensor data monitored by government or a company) and given moral agency. Each represents different risks but each needs to be considered.
The reduction in cognitive skills where a person loses skill at driving, might be regarded as acceptable and requiring minimal legislation. The surveillance potential using vehicle sensor information would prompt consideration of the governance needed around security and privacy. The moral dilemmas surrounding safety (e.g. should the vehicle be programmed to prioritise the safety of its occupants over others) might lead legislators to determine that self-drive vehicles should never be fully autonomous on the grounds that assigning liability could be problematic. Used in this way, rather than creating bias, the taxonomy allows different risks to be assessed and appropriate governance to be developed.
Freedom to choose
There are many uses of AI where we have freedom to choose and to exercise self-control, either by moderation or abstinence, whether they be digital assistants such as Google Home, Satnav systems, browsers or tools in our workplace. The challenge arises when we have no control or choice over whether we’re subjected to applications, such as facial recognition, emotion detection or an Automated Decision Support System (ADSS). This will be the case when they’re used by the state in the public sphere without our consent. The harms principles would at least alert stakeholders in the deployment of such systems whether freedom of choice should be built in as a governance principle, for example, a patient could be given the opportunity to opt out of the use of a medical diagnostics tool.
The debates continue about what applications should be totally banned because of the risk associated with them. The trade-off is usually economics and efficiency yet, as the authors of IEEE Global Initiative on the Ethics of Autonomous and Intelligent Systems, Ethically Aligned Design noted, this shouldn’t be the only criteria. I would argue that humans should retain responsibility for decision-making in areas where the lives of others are affected. For governments and corporations that means that we should not entrust to an algorithm the responsibility to make risk judgements about parole, reoffending, children potentially at risk, visa applications and many more areas.
it is by no means guaranteed that the impact of these systems will be a positive one without a concerted effort by the A/IS community and other key stakeholders to align them with our interests.
IEEE Ethically Alligned Design, 2019
References
A. Jobin, M. Ienca, and E. Vayena. The global landscape of AI ethics guidelines. Nature Machine Intelligence, 1(9):389– 399, 2019.
A. Renda, Artificial Intelligence: Ethics, Governance and Policy Challenges, Brussels: Centre for European Policy Studies, 2019, pp. 56–57.
IEEE Global Initiative on the Ethics of Autonomous and Intelligent Systems, Ethically Aligned Design: A Vision for Prioritizing Human Well-being with Autonomous and Intelligent Systems, IEEE, 2019,
J. B. Peckham, “An AI Harms and Governance Framework for Trustworthy AI,” in Computer, vol. 57, no. 3, pp. 59-68, March 2024, doi: 10.1109/MC.2024.3354040.
J. Peckham, Masters or Slaves? AI and the Future of Humanity, IVP: London, 2021, p. 187. (book)